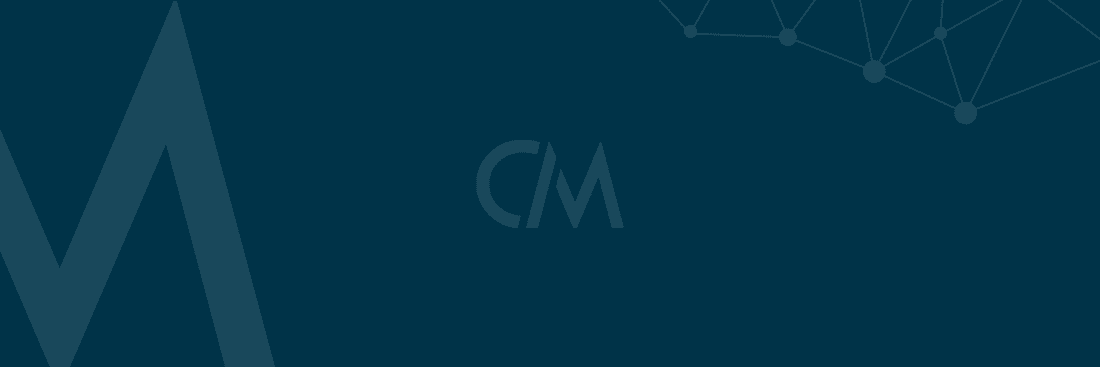
Weekly Feature
Cryptoasset Valuation Research Primer, Part 1
by Kevin Lu and the Coin Metrics Team
Cryptoassets represent a significant innovation in the evolution of money and the modern financial system. Valuing cryptoassets remains very much an open question. Foundational concepts on which a formal discipline of crypto valuation can be built are only beginning to be established. Without a firm anchor to existing methods of asset valuation, we have seen intense experimentation over the past 10 years.
We conducted a comprehensive literature review to identify all major facets of cryptoasset valuation research that has been conducted so far. All methods were considered, from theoretical valuation frameworks, to empirical valuation models, to novel indicators that have application to valuation.
In short, we are interested in all research that can be used to understand the current value of cryptoassets, estimate the value of cryptoassets, or predict future values of cryptoassets. All publication mediums are considered, regardless of pedigree, from forum postings to academic journals. The most salient articles from both academic and industry researchers are included.
In this piece, we explore six main categories of crypto valuation research: equation of exchange, discounted future utilities model. Metcalfe’s law, price regression models, cost of production models, and asset bubble identification. In an upcoming Weekly Feature, we will review factor investing, transfer value-based ratios, realized capitalization, and the emerging field of UTXO analysis as Part 2 of our cryptoasset valuation research primer.
Equation of Exchange
Fisher’s equation of exchange embodies a strand in the literature that has been influential in the field of token design. Originally designed to explore the relationship between money supply and price level in the field of monetary economics, it has been applied to the field of cryptoasset valuation and currently stands as one of the most widely explored theoretical frameworks.
The core idea is simple and intuitive: the equation of exchange is the relation MV = PQ, where M is the nominal amount of money, V is the velocity of money, P is the price level, and Q is the index of real expenditures. This is succinctly explained in Wang (2014).
The equation is adapted to take into account the unique aspects of cryptoassets such as Bitcoin (BTC). First, all quantities are defined in units of fiat currency, setting P equal to 1. M is defined as the number of cryptoasset in existence multiplied by the price of a single unit of the cryptoasset (i.e. the market capitalization). Q is the amount of value transferred across the network. And the interpretation of V remains unchanged.
The implication of this model is that the value of a cryptoasset has an inverse relationship with velocity -- that is, high levels of velocity lead to lower cryptoasset valuations. Wang (2014) finds that the price of BTC is determined solely by the likelihood that BTC will be saved. Ciaian, Rajcaniova, and Kancs (2015) empirically test the impact of supply and demand factors on BTC price, including velocity (which they proxy by using BTC days destroyed), and find that they have a significant impact.
BTC velocity has decreased in recent years as price has increased, which fits with the equation of exchange model. The following chart shows adjusted velocity (i.e. velocity computed using adjusted transfer value which filters out self-sends and spam transactions) of the one-year active supply (i.e. supply that has been transacted at least once within the last year) of BTC.
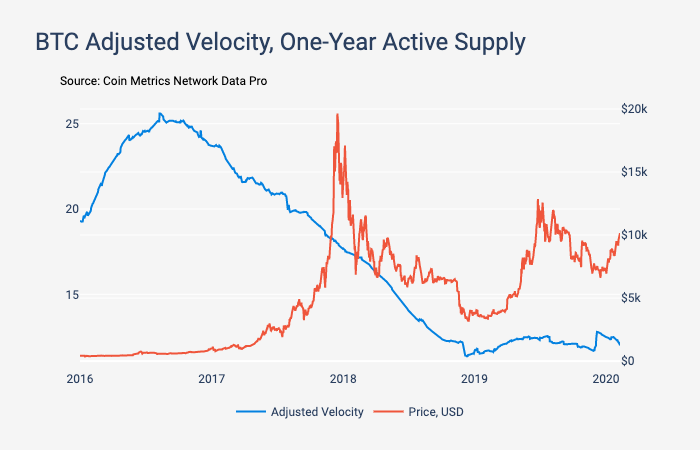
The literature’s fixation on velocity continues in Buterin (2017) and Pfeffer (2017), two seminal articles that apply the equation of exchange to the valuation of utility tokens. Both researchers posit that utility tokens are susceptible to extremely high levels of token velocity because users only procure small amounts of tokens to use a service provided by the network and providers of the service immediately sell any tokens they receive. Buterin (2017) concludes that the value of a cryptoasset “depends crucially on the holding time” of the token and argues for token sinks -- mechanisms which reduce the token supply or token velocity (for example, transaction fee burning). Pfeffer (2017) concludes that token velocity could be high at equilibrium, and the value of a utility token will converge to a low level that is a fraction of the actual cost of the computing resources needed to maintain the network.
ETH adjusted velocity of one-year active supply increased leading up to the 2018 price peak, and has since leveled off. ETH’s 2017 rise in velocity also coincides with the ICO boom and the rise of utility tokens, which has since subsided. ETH’s velocity appears to be relatively in line with ETH price.
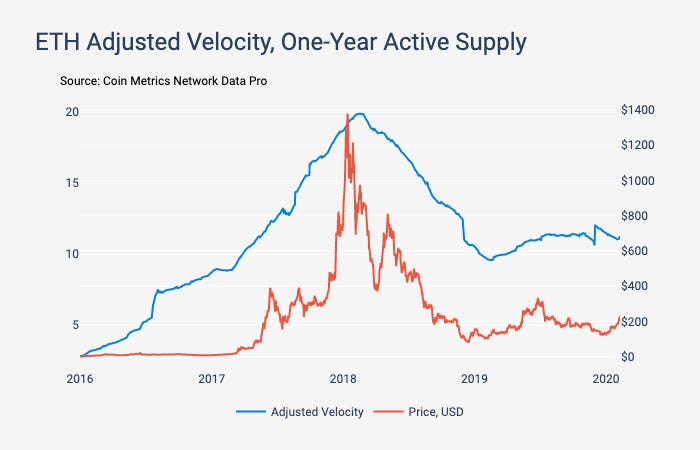
Samani (2017) and Samani (2018) present ideas on token economic models which address the “velocity problem” to allow a utility token to accrue value. Burning and staking models are considered. Collectively, the articles published by industry researchers under this topic in 2017 and 2018 were strongly influential in the field of token design.
The high velocity thesis has garnered its fair share of criticism, however. Evans (2018) presents one of the most salient criticisms, suggesting that previous frameworks view token velocity as an exogenous variable (a value determined outside the model) that can be tuned to a desired level through token mechanism design. Instead, Evans (2018) models velocity as endogenous to the model and a function of PQ. Koralewski (2018) makes similar criticisms regarding the exogeneity of velocity. Weber (2018) challenges the entire foundation of the velocity thesis by arguing that the equation of exchange has been mathematically misapplied and presents two correct applications. Similarly, Locklin (2019) presents a devastating criticism of the equation of exchange and argues that commonly held conclusions regarding token velocity and optimal token design are false.
Despite its criticisms, the equation of exchange remains one of the most widely adopted frameworks for token valuation. In the coming years, as winners and losers emerge in the utility token space, we expect more empirical research to be done in this area to test the token velocity thesis.
Discounted Future Utility Models
The discounted future utility family of models takes inspiration from valuation using a total addressable market approach, discounted cash flow analysis, and the equation of exchange. It represents a significant extension of the theory presented in equation of exchange research by advancing an empirical model to estimate the value of utility tokens. Token velocity, among other assumptions, is one of the key parameters in the model. This family of models most closely resembles a discounted cash flow model found in traditional finance, but adapted to the unique characteristics of cryptoassets.
Burniske (2017a) represents the first significant contribution to the literature by introducing a model to value a hypothetical utility token. Key determinants of the model are the token’s supply characteristics such as the number of tokens in circulation, the total size of the market that the utility token is used to purchase services from, the adoption curve of the network, token velocity, and the discount rate. Winton (2017) introduces a similar model with many of the same model parameters but also allows for different return expectations from different cohorts of investors.
Burniske (2017b) introduces the terms “current utility value” and “discounted expected utility value”, and presents a theoretical framework for how value derived from the two sources fluctuates throughout the lifecycle of an utility token.
The application of discounted future utility models remains an area where considerable empirical research can be conducted. Functional networks upon which utility tokens are built are still under active development, and a few significant projects are seeing meaningful amounts of activity. Modeling the value of utility tokens with novel token designs such as burning, discounting, and staking also remain an active area of experimentation and research.
Metcalfe’s Law
Metcalfe’s law states that the value of a communications network is proportional to the square of the number of connected users of the system. The foundation of this law is in the mathematical relationship that each user of a communication network can make (n - 1) connections with other users. If each connection is considered equally valuable, the total value of the network is proportional to n (n - 1) / 2, which asymptotically approaches n2. Metcalfe’s law has been successfully applied to the valuation of social networks. As an example, Zhang, Liu, and Xu (2015) empirically test Metcalfe’s law and several other network effect laws by using data from Tencent and Facebook and find that Metcalfe’s law fits better than competing laws.
Application of Metcalfe’s law to valuing cryptoassets is straightforward and was first conducted in gbianchi (2014), where the key insight was made to define a user of the Bitcoin network as the number of addresses with zero balance, a proxy chosen after backtesting and considering other alternatives. A formula to predict the price of BTC based on the square of the number of addresses with zero balance is presented. The article also made a significant contribution to another thread in the literature by introducing the idea of tracking the number of addresses between certain balance thresholds.
Alabi (2017) tests Metcalfe’s law on BTC, Ethereum (ETH), and Dash (DASH), and illustrates how deviations from predicted values can be used to identify asset bubbles. Peterson (2018) applies Metcalfe’s law using an alternative representation for users: the number of wallets from blockchain.info. The model is used to identify a period of suspected market manipulation in 2013. Franek (2018) tests Metcalfe’s law and competing laws on BTC and ETH, and introduces a price-to-Metcalfe ratio to identify periods of over or undervaluation. Kalichkin (2018) combines predictions from Metcalfe’s law with predictions from Odlyzko’s law and similarly introduces a price-to-Metcalfe ratio.
The application of Metcalfe’s law to cryptoassets touches upon another emergent element in the literature: the study of the number of users of a particular cryptoasset. While the transparency afforded by blockchain ledgers allows for many candidate proxies to represent the number of users, we still lack the clarity of a concept similar to daily active users, a common metric used to track the usage of internet applications. The mapping of on-chain activity to real world entities and individuals is still unclear and an active area of research. As more work is done in this adjacent area, a more precise application of Metcalfe’s law to valuing cryptoassets is possible.
Price Regression Models
Price regression models refer to an approach to cryptoasset valuation where price is regressed on another variable, typically time (or a variable that is a function of time). The defining characteristic of this approach is that predicted price values can be generated far into the future, with some models predicting prices that are unimaginable today. While some practitioners may dismiss this family of models because of its simple approach, we believe it is a mistake to ignore them entirely -- early models have had remarkably accurate out-of-sample results, have reliably identified historical periods of over and undervaluation, and still receive considerable attention from market participants.
Trololo (2014), building on early prior research in the field of cryptoasset valuation, represents the genesis of the price regression family of models. Using a model that regresses price on the natural log of time, Trololo (2014) was able to predict the date that BTC would reach $10,000 with an error of only a few days at a time when the current price was $275. Residuals from the model are used to identify periods of over and undervaluation.
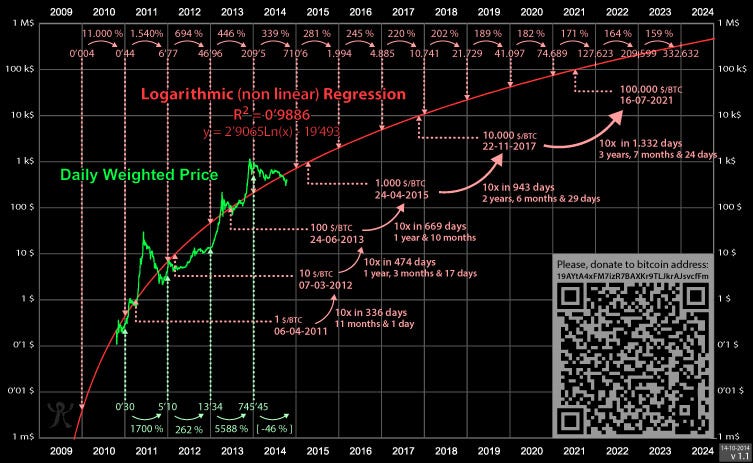
Awe & Wonder (2018) uses a similar approach with updated data and provides a prediction for the low of the market cycle with very good out-of-sample accuracy. Burger (2019) presents various price regression models using subsets of the data to test for robustness of fit.
PlanB (2019) was the next significant advancement in the field and represents one of the most impactful articles in cryptoasset valuation research. Taking inspiration from Ammous (2018), PlanB (2019) posits that there is a relationship between BTC’s value and its stock-to-flow ratio. Stock-to-flow ratio is defined as the inverse of annualized supply issuance, and represents BTC’s scarcity and suitability as a store of value. A test using empirical data finds co-integration between market value and stock-to-flow ratio with high goodness of fit. The model predicts a BTC price of $55,000 after the next halving in May 2020.
Price regression models have had remarkable success in prediction and adoption. But their legitimacy rests on the assumption that BTC, in particular, will continue on a path to a long-term equilibrium where it becomes a global store-of-value asset, comparable to gold. The next few years will generate additional data which can be used to test the primary assumptions of these models, such as the co-integration between BTC’s value and its stock-to-flow ratio.
Cost of Production Models
Cost of production models touch on a strand in the literature which quantify the costs of mining in order to value cryptoassets. Such an approach is intuitively straightforward and is rooted in classical economics where, for example, Adam Smith introduced the concept of a natural price and market price for commodities. The natural price is the price level that is equal to the cost of the various factors of production necessary in producing a commodity. The market price is the actual price that the commodity is sold. Smith argues that the natural price is the central price to which all commodities are continually gravitating.
Satoshi Nakamoto succinctly explains the foundational logic behind this approach: “The price of any commodity tends to gravitate toward the production cost. If the price is below cost, then production slows down. If the price is above cost, profit can be made by generating and selling more. At the same time, the increased production would increase the difficulty, pushing the cost of generating towards the price.”
The application of a cost of production model for valuing BTC occurred out of necessity as early as 2009, the first year of BTC’s existence. New Liberty Standard, the first website to offer a BTC exchange, was also the first to establish an exchange rate for BTC -- the first published exchange rate in October 2009 was 1,309.03 BTC to one U.S. Dollar. In the absence of an established market, the administrator of the website calculated the exchange rate using a simple model that approximated the cost of electricity needed to mine BTC.
Hayes (2015) represents the first serious treatment of the subject and offers a model based on the cost of electricity, the efficiency of miner technology, the market price of BTC, and the difficulty of mining. Hayes (2016) conducts a cross-sectional analysis on 66 cryptocurrencies and finds that a cryptoassets value can be explained by mining difficulty, the rate of supply issuance, and the type of mining algorithm used.
The Cambridge Bitcoin Electricity Consumption Index, using miner hardware performance in Bevand (2017) provides a lower bound, upper bound, and best guess estimate for the electricity consumption of BTC. Edwards (2019) presents a model where BTC’s value (referred to as its energy value) is a function of energy input, supply issuance, and the fiat cost of energy input. Predicted values from the model using empirical data find good fit.
Understanding BTC’s cost of production has an important impact on miners who have a role in the formation of asset bubbles-and-crashes due to their procyclical behavior. The economics of mining and conceptual model for estimating the cost of production of cryptoassets is now well understood. Further advancements in this field are likely to be in gathering more accurate empirical data upon which these models are based on.
Asset Bubble Identification
The tendency for speculators to create bubbles in financial assets is deeply rooted in human psychology. Cryptoassets, without a firm anchor to traditional methods of asset valuation, are particularly susceptible to bubbles and bubble-and-crash cycles have happened several times in BTC’s short history. The size and frequency of bubbles in cryptoassets invites the application of bubble detection techniques, first developed in traditional financial assets.
Cheah and Fry (2015) is among the earliest articles to apply established bubble detection techniques to BTC. Using a variety of bubble detection models, it finds empirical evidence that BTC is prone to substantial speculative bubbles. Using a recently developed detection technique, Cheung and Su (2015) finds many short-lived bubbles and three large bubbles in the period between 2011 to 2013. Wheatley, Sornette, Huber, Reppen, and Gantner (2018) presents a generalized version of Metcalfe’s law which does not require network value to grow proportionally to the square of the number of users to model the fundamental value of BTC. Deviations from predicted values from this model are considered bubbles and they are formally tested using a textbook bubble detection technique. Four bubbles are detected and an ex ante prediction is provided that performs well out-of-sample.
Conclusion
The Dutch East India Company, founded in 1602, was the first corporate entity to issue bonds and shares to the public, and in doing so became the world’s first formally listed public company. It then took a period of over 300 years for the necessary foundational concepts to be developed until the formal discipline of equity valuation was established in the 1930s. With cryptoassets, we stand on the shoulders of giants, and substantial progress has been made over the past 10 years in the emergent field of cryptoasset valuation research. Existing valuation methods across many disciplines are being adapted to suit cryptoassets. At the same time, unique cryptoasset-specific methods are being actively developed. Foundational concepts are only beginning to be established and many concepts likely remain undiscovered.
In an upcoming issue of State of the Network, we continue with Part 2 of our comprehensive literature review and cover other significant advances in cryptoasset valuation research. Factor investing, transfer value-based ratios, realized capitalization, and the emerging field of UTXO analysis are among the topics covered. We also share our outlook on the most promising future directions of valuation research.
Network Data Insights
Summary Metrics
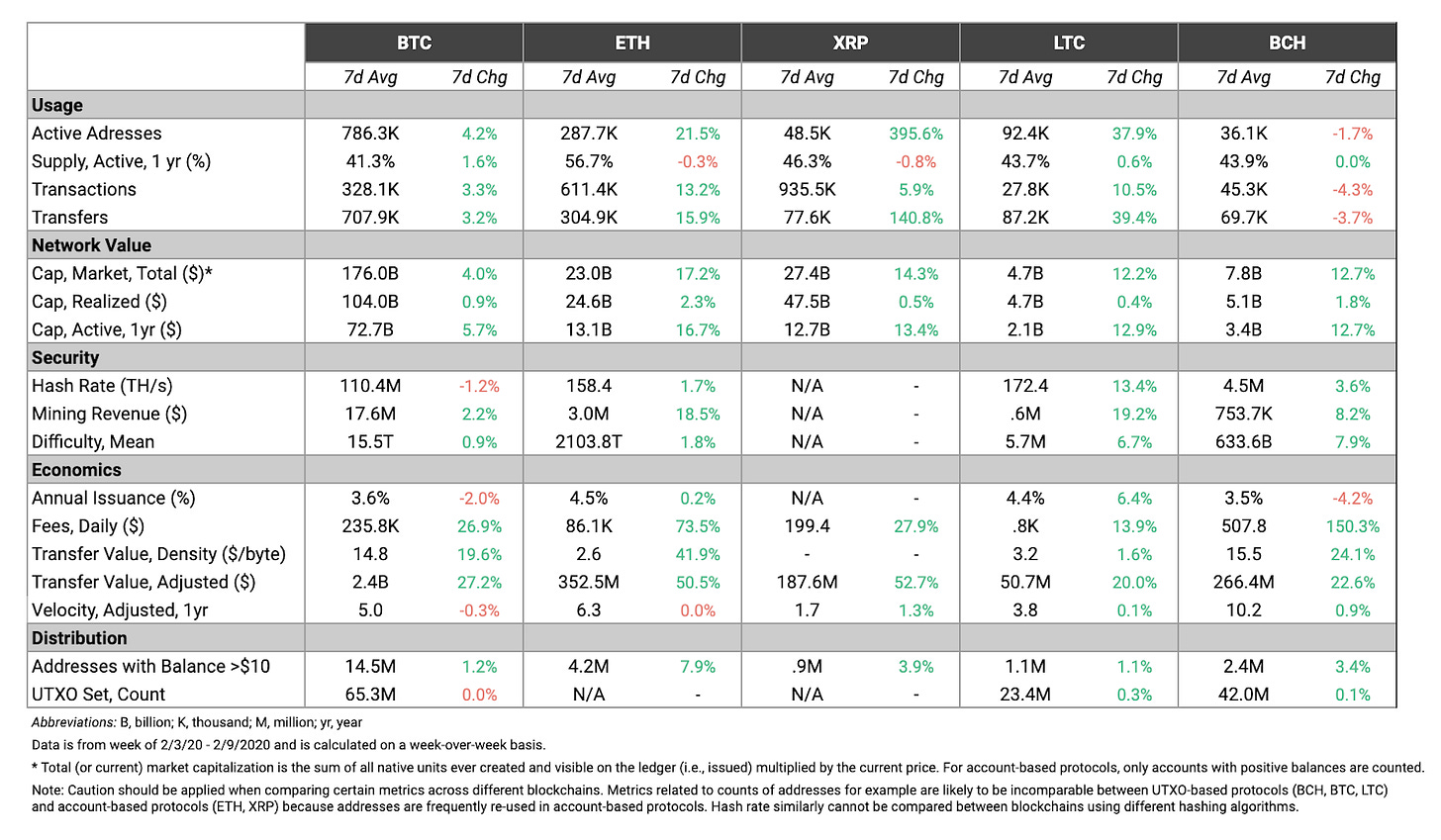
The major cryptoassets had another strong week, continuing a hot start to 2020. BTC was up across most metrics, but ETH was up even more in almost every category. Although BTC led the way for much of 2019, we have now seen ETH and many smaller-cap assets outperform BTC at the start of 2020. ETH posted five days of consecutive positive returns from February 5th through February 9th, which has only happened 21 times in ETH’s history.
ETH also outpaced BTC this past week in usage growth. ETH active addresses grew by 21.5% and transaction count grew by 13.2% week-over-week, while BTC active addresses grew by 4.2% and transaction count grew by 3.3%.
Network Highlights
Velocity of one-year active supply (i.e. the supply that has been transacted at least once within the last year) of stablecoins is near all-time highs. The following chart shows the average velocity of one-year active supply for the following stablecoins: Tether (Omni, Ethereum, and Tron), Paxos, USD COIN, DAI, TrueUSD, and Gemini Dollar. Increasing velocity suggests that stablecoins are changing hands more often, which suggests they are potentially increasingly being used as a medium of exchange.
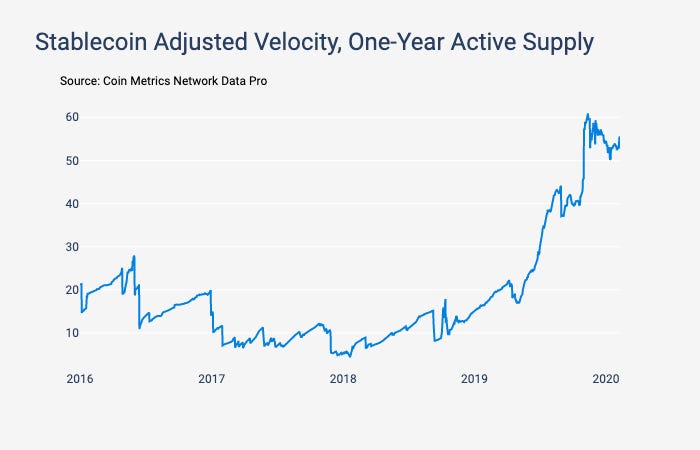
Tezos (XTZ) realized cap has been growing faster than most other assets over the start of 2020. Realized cap can serve as a proxy for investor cost basis. Although BTC realized cap growth outpaced XTZ over most of 2019, XTZ has surged past BTC in 2020.
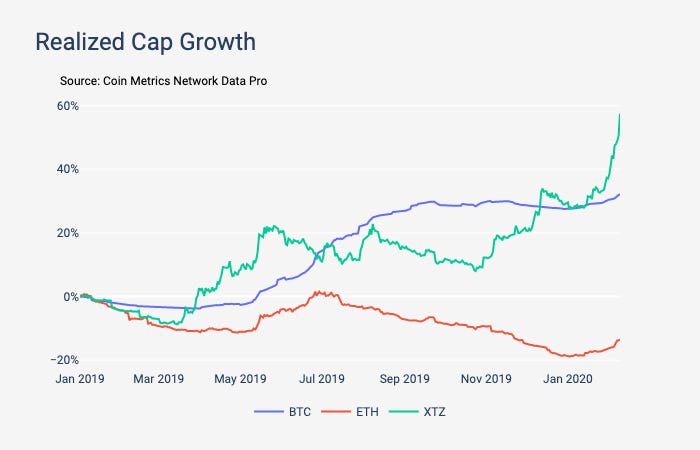
Market Data Insights
A large move widely expected by market participants has not yet materialized. Open interest on BitMEX’s XBTUSD contract remains high and the difference between realized and implied volatility in the options market remains elevated. A sharp drop in BTC’s price from $10,100 to $9,800 over the weekend caused some liquidations in the XBTUSD contract but failed to trigger a cascade of further liquidations.
Market breadth is quite positive with nearly all assets in our coverage universe holding onto strong weekly gains. The recent pattern of other assets outperforming BTC has continued with BTC only gaining +9%. ETH (+21%) is a notable performer, along with XTZ (+41%) reaching an all-time high, and Binance Coin (+31%).
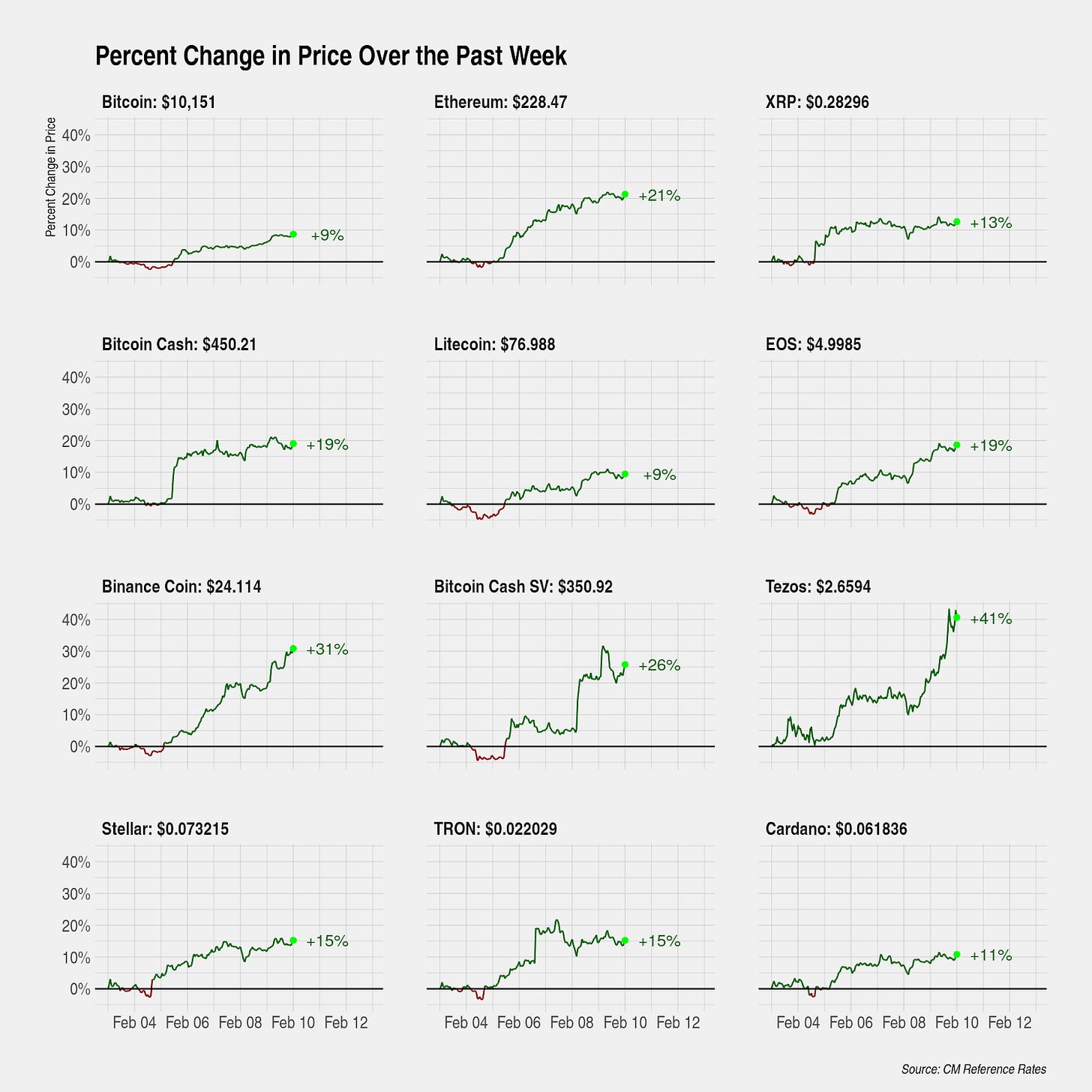
Ethereum Classic (+3%), Dash (+12%), and ZCash (+7%) have been significant outperformers over the past several weeks but only saw relatively modest gains this week. NEM (+35%) is now a project to watch -- it had one of the strongest weekly gains and is up +98% over the past month.
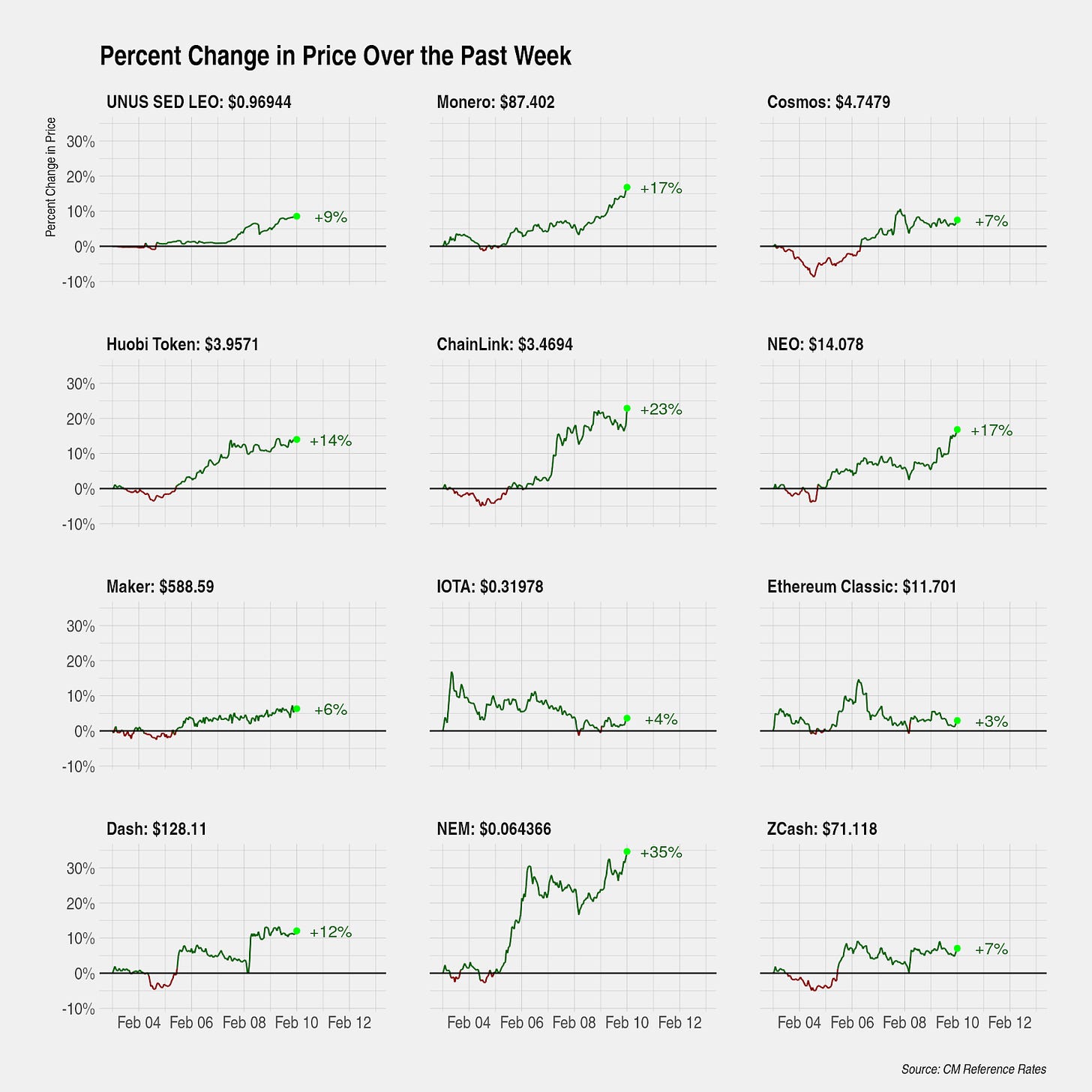
CM Bletchley Indexes (CMBI) Insights
This week, cryptoassets and indexes continued their impressive start to the year. Even-weight indexes performed strongest again, demonstrating the market wide nature of this week's performance and the strength of some of the lower value assets in each index.
Of the market cap weighted indexes, the Bletchley 20 (mid-cap) and Bletchley 40 (small-cap) continued to perform best, providing weekly returns of 19% and 18% respectively. Both these indexes are yet to have a negative performing week in 2020.
All indexes outperformed the CMBI Bitcoin Index for the week, with the Bletchley 20 Even and the CMBI Ethereum Index performing the strongest, returning 24% and 21% respectively.
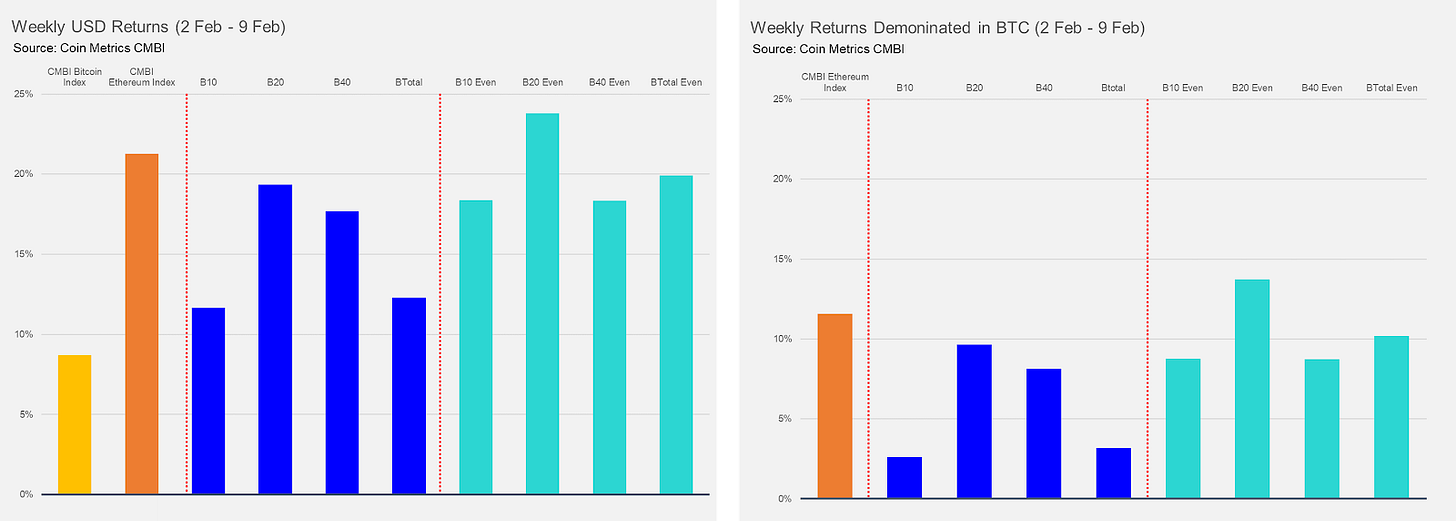
Coin Metrics Updates
This week’s updates from the Coin Metrics team:
Coin Metrics is hiring! We recently opened up 4 new roles, including Blockchain Data Engineer and Data Quality and Operations Lead. Please check out our Careers page to view the openings.
As always, if you have any feedback or requests, don’t hesitate to reach out at info@coinmetrics.io.
Subscribe and Past Issues
Coin Metrics’ State of the Network, is an unbiased, weekly view of the crypto market informed by our own network (on-chain) and market data.
If you'd like to get State of the Network in your inbox, please subscribe here. You can see previous issues of State of the Network here.
Check out the Coin Metrics Blog for more in depth research and analysis.